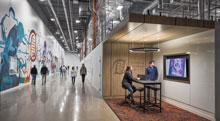
Integrating AI: How to Incorporate Artificial Intelligence Into the Laboratory of the Future
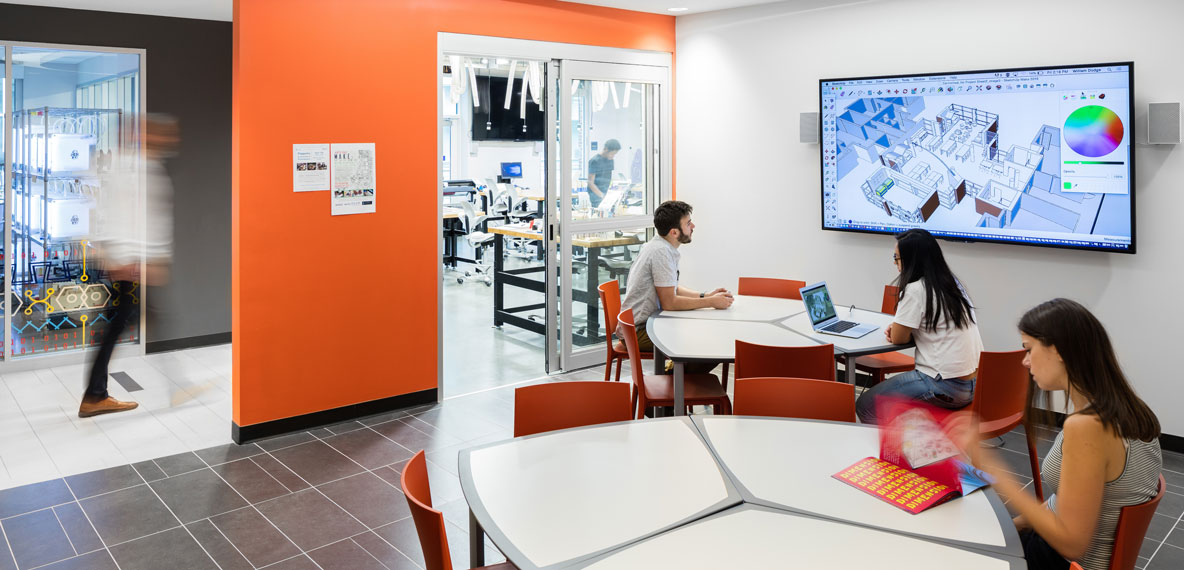
Artificial intelligence (AI) promises to play a significant role in how science and technology research environments are planned, constructed, and operated in the near and distant future. AI, along with its subset, machine learning (ML) will drastically affect how research environments are transformed, ultimately benefiting human health. Although AI offers numerous advantages, it is not without its challenges, risks, and issues. Continuous human oversight, data influence, and ethical concerns are a few elements to consider and address when integrating AI into research facilities and programs. In addition, it’s important to examine AI as an instrument to enhance, rather than replace, the skill and expertise of researchers, managers, and owners who are integral in human health research’s ethical and responsible conduct.
Weighing the benefits
AI brings a host of potential benefits to the laboratory of the future. For example, AI can offer increased effectiveness, efficiency, and precision, minimize the need for animal testing, enable the delivery of more personalized medicine and healthcare, and aid the drug discovery process.
These capabilities help predict and prevent potential health crises. The efficiency and accuracy that AI and ML provide through predictive modeling are especially beneficial. Predictive modeling is a mathematical process that assesses patterns within a given dataset to help predict future events or outcomes. Predictive modeling relies on ML and data mining to forecast likely outcomes, facilitated through the detailed investigative analysis of current and historical existing data that thorough examination has confirmed as accurate. AI and ML can harness the tremendous analytical power of the world’s most advanced computer systems and their ability to process vast amounts of data in seconds.
For instance, AI-related programs work information on linked healthcare networks worldwide, using complex algorithms designed to examine and cross-reference interrelated material to foresee areas at risk for emerging diseases, viruses, or other health-related pandemics. As such, healthcare professionals, government entities, and social welfare organizations can prepare ahead of time to establish preventive programs, develop therapeutics, and take measures to defend against an outbreak before it becomes unmanageable.
AI can also enable new approaches to research discovery. For instance, AI and ML are capable of fast and effective data analysis and pattern recognition while automating parts of literature review, providing data visualization and interpretation, and generating hypotheses. These technologies can also expedite drug discovery, performing computer simulations to predict drug effectiveness and targeting promising compounds. In addition, helping research teams focus on effective therapeutics reduces the need for animal testing.
AI-driven robotics and automation increase lab efficiency, rapidly and accurately testing thousands of samples. This can expedite the U.S. Food and Drug Administration approval process by shortening the time required to collect and analyze the necessary data to demonstrate a drug’s safety.
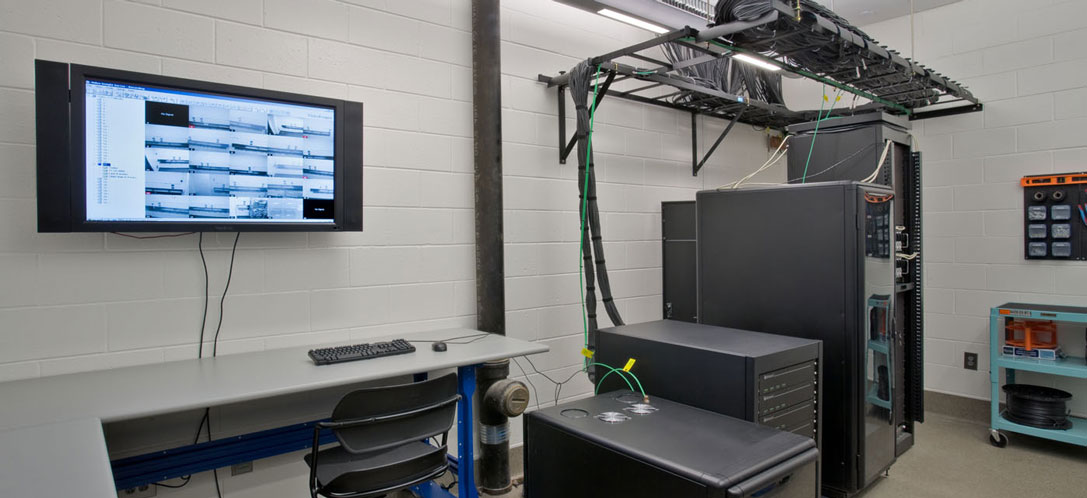
Providing space that includes data storage and retrieval systems is critical when incorporating artificial intelligence and machine learning.
Considering the challenges
Integrating AI and ML into the laboratory environment involves addressing concerns, including financial considerations. AI solutions can be costly, depending on their complexity and other factors, and an organization must carefully weigh that cost before implementing AI in the lab environment.
From an infrastructure standpoint, fast and reliable high-speed internet connectivity is perhaps the most essential element required of a research facility wanting to leverage the advantages of AI and ML. It is critical for designers and contractors to plan for the incorporation of equipment and technology that supports the ability to retrieve and disperse large quantities of information as quickly as possible under strict rules and secure conditions. Data capacity and management protocols are also vital, as they ensure that information is maintained and readily available.
Regarding the workspace, space types and furniture that enhance collaboration and interaction, support and sustain contemplation, and provide quiet meditation are also vital. Employees using this space include those who analyze complex data sets and compare them to research results and employees who develop complicated algorithms. Incorporating elements that allow these employees to perform their duties unhindered include multimedia functions, visualization systems, and equipment to encourage remote communication and interaction with sister institutions and organizations. Uninterrupted power supply to computers and storage mainframes and generator backup power to equipment that helps ensure continuity in the access to data is also crucial.
Quick and reliable access to the data generated by AI and ML demands a robust information technology system within the facility. This encompasses powerful computer mainframes, adequate, reliable Wi-Fi routers, and internet access points that are secure from outside influences, redundant storage devices with multiple terabytes of capacity, and processing power.
These elements also require readily available space for the equipment and IT backbone and to house those with the expertise to manage and maintain the systems. These systems generate significant heat, necessitating powerful cooling components and HVAC equipment to ensure smooth function and longevity. There’s also the data that the new caging systems and telemetry devices obtain, increasing the need for large-capacity computing power, information storage, and distribution equipment. Ready access, including generators and uninterrupted emergency power supply, is also required.
It’s critical for animal holding and research procedure spaces to be flexible and adaptable and include more advanced technology to quickly adjust to different animal species as research objectives, protocols, and directives evolve to address the latest investigative approaches that AI and ML develop. These aspects include animal holding spaces that integrate trench or floor drains, hose reels and waterproof finishes, lighting fixtures, and modulating airflow devices that can easily change the space from positive to negative. Procedure rooms should have mobile casework that can be moved out to convert the space to a temporary holding space and back again. Environmental controls that monitor the particulate output of a space and regulate the exhaust system based on occupancy needs are paramount.
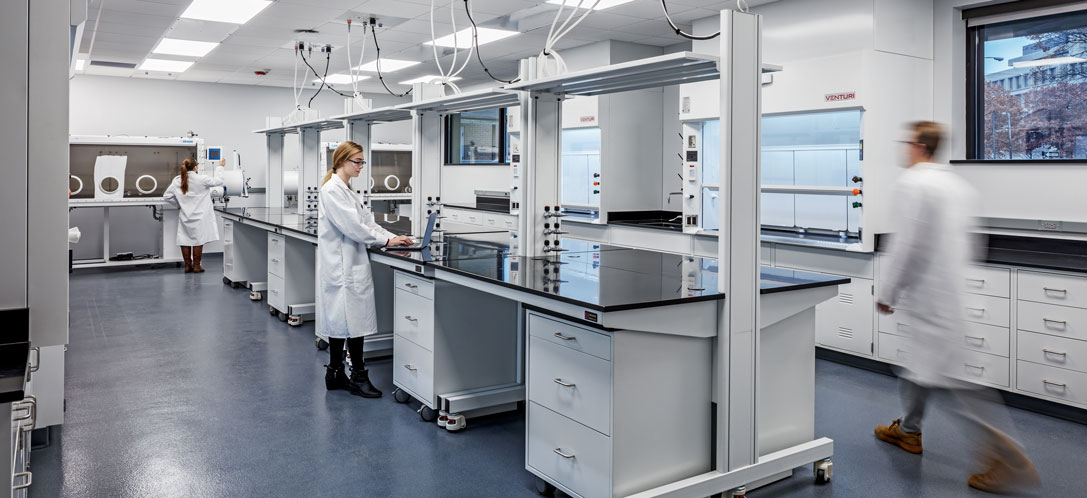
Mobile, flexible casework with ceiling-mounted utility panels supports the ability of a research lab to change quickly to address adjustments in research objectives and the incorporation of new equipment.
Significant challenges also exist regarding the quality of the data used to inform decisions. This is a potential issue wherever AI and ML are employed, including in the laboratory. Consider the recent launch of Google’s Gemini AI program, which produced questionable conclusions, if not blatantly inaccurate. Like much of the information found on the internet should be vetted for accuracy and lack of prejudice, so too must the results of AI be evaluated and checked for accuracy by individuals knowledgeable and informed about research results when dealing with science and technology-related data.
In other words, bias in the data results in bias in the outcome. As such, the precision of the results is under suspicion without a thorough validation process that can verify the results. Interpreting that data is a complex process, which creates the potential for misinterpretation. This underscores the importance of ensuring that highly knowledgeable subject matter experts analyze the outcome.
The bias issue is an example of the ethical concerns associated with AI and ML. One recent article cited data bias as the most critical challenge surrounding AI use, noting that “AI systems are only as good as the data they’re trained on, so objective data curation becomes paramount.” In the same piece, AI experts underscored the need to prioritize and standardize “rigorous testing and continuous monitoring.”
Privacy issues abound, too. In addition to data accuracy, the protection of intellectual property created by individuals or organizations who have expended extensive amounts of time and resources developing their theories and associated conclusions is one of the most challenging aspects of implementing AI and ML into the research. Giving credit where credit is due is not always easy, especially when the results come from many different sources with varying degrees of input. The large amounts of data that AI collects and relies on to make decisions and predictions also raise concerns about how that data is used and by whom.
Ultimately, AI and ML depend heavily on accurate, unbiased data to be effective and productive. These tools can augment, support, and sustain processes and procedures currently performed by individuals rather than replace, discard, or eliminate them. It’s imperative for science-based institutions and organizations to incorporate the infrastructure required to sustain and facilitate this advanced technology and processes into their research programs to take advantage of the many benefits the technology offers.
This article was originally published by Labcompare.
Let’s get to work. Complete our contact form today.
Author
Content Type
Published Articles
Date
August 05, 2024
Market
Practice
Laboratory Planning
Topic
Laboratory Design
Technology